
The robustness of this modelling approach is thoroughly manifested through its numerous advantages over other linear and nonlinear methods for measuring market linkages. For instance, under (cross)market linkages or co-variations, the wavelet coherence approach has been found useful due to its ability to provide insightful information on dynamic market behaviour across time and over different investment scales. The application of wavelet methods to financial market analysis has been prominent in recent literature. Imhotep Paul Alagidede, in Research in International Business and Finance, 2019 2.2 The wavelet coherency framework The study revealed strong coherence at lower frequency space. The authors used wavelet coherence analysis to examine the linkages between FTSE100, DJIA30, Nikkei225 and Bovespa stock exchanges. A change in pattern was detected in the year 2006, wherein coherence at higher frequencies was revealed. High coherence was observed at lower frequencies. The authors studied the level of integration of 22 emerging stock markets with the US market. The strength of this dependency was found to vary across countries. Strong dependency was found at lower frequency space. The authors studied the multi-scale dependencies between stock market and oil price movements within the Gulf Cooperation Council (GCC) countries. High coherence was observed at medium scale during the sub-prime crisis and at the lower scale during the European Debt Crisis. The authors probed the inter-dependency of 13 Asia-Pacific stock market with the European and US stock market using wavelet coherence approach and revealed significant variations in the co-movements during the crisis period. In the frequency domain, high dependency was observed at low frequency region and a modest dependency at the higher frequency space. High coherency was detected during the 2007 crisis period. The strength of co-movement was found to vary across time and frequency. The authors probed the global and regional co-movement of MENA stock markets using wavelet coherency. The authors used wavelet squared coherence to study the dependencies among the stock market returns of Gulf Cooperation Council (GCC) countries and found strong evidence of scale dependencies, particularly during the 2007 crisis. Their finding was further validated by several researchers, some of whose works are sketched in Table 4. The authors found strong co-movements among the financial markets at lower frequency regions and not at higher frequency space, which indicated that the advantage of cross-market diversification is lower for long term investors relative to their short-term counterparts.

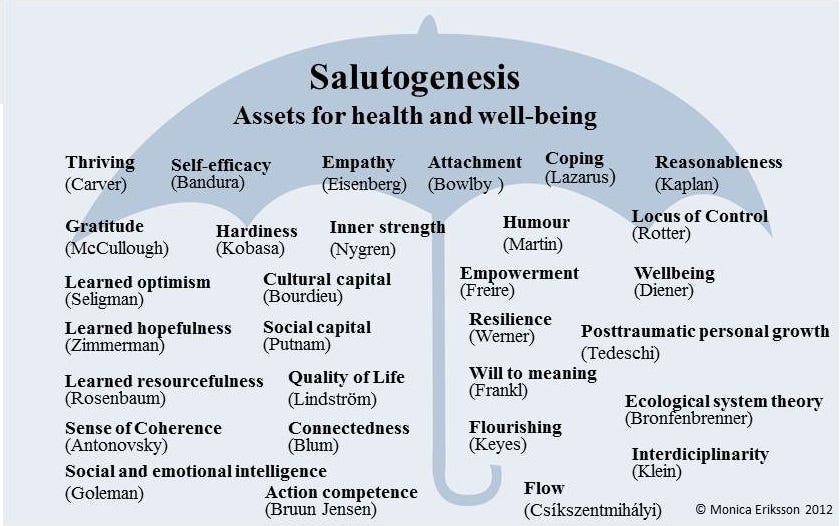
Following their work Rua and Nunes introduced wavelet coherence in the study of inter-linkages between financial markets. were the first to introduce wavelet coherence in analyzing the impact of change in interest rate on macroeconomic variables. It was in the year 2008, that this methodology migrated into the domain of economics. The method was pioneered by Torrence and Compo and Torrence and Webster and has been extensively used since then in different scientific domains, ranging from medicine, to astrophysics and geophysics. In comparison to the wavelet correlation analysis, wavelet coherence can effectively identify regions of high co-movement in the time–frequency space.
#Coherence explained series#
Wavelet Coherence a bi-variate framework used to study the interaction between different time series and their evolution over a continuous time and frequency space. The methodology of wavelet coherence has gained immense popularity over the last few years in the domain of finance and economics. Note: An example of a wavelet coherence graph is provided in Fig. More about the interpretation of a wavelet coherence graph and the axis and color representations are provided by Uddin et al. The thick black contour line is the 5% significance level. The direction left-down indicates lagging of the variable. The direction left-up indicates leading of the variable. Īrrows pointing to the left indicate out of phase variables.The direction right-down indicates leading of the variable. The direction right-up indicates lagging of the variable (e.g., uncertainty). Īrrows pointing to the right indicate perfectly phased variables.Īrrows indicate the direction of comovement between variables.In page 36, they note useful technical interpretations: (2018) is very important because it is among the very few ones that are so extrovert and explain the way the visual findings from a cross- wavelet coherence and phase diagram are explained.
